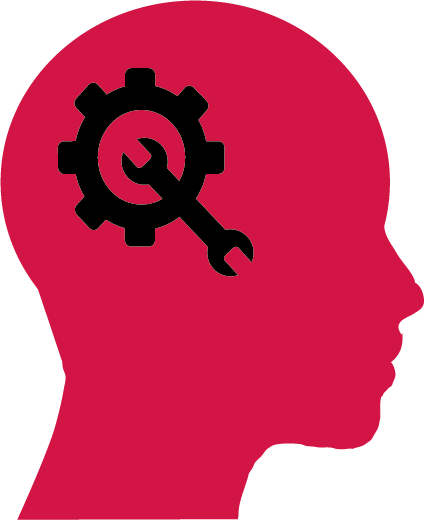
Understanding Mental Illness: Computational Psychiatry
Translating advances in neuroscience into benefits for patients with mental illness presents enormous challenges because it involves both the most complex organ, the brain, and its interaction with a similarly complex environment. One promising approach to this challenge is computational psychiatry. This nascent field integrates diverse computational methods with multifaceted data to enhance our understanding, prediction, and treatment of mental illness (Petzschner et al, 2017).
In the lab, we have developed experiments and complex models to capture various aspects of cognition, spanning perception, learning, and action selection in clinical populations. Specifically, we study the origins of perceptual biases in Autism Spectrum Disorder (Schneebeli et al., 2022), and the role of action selection and beliefs about agency for the development of compulsions in Disordered Gambling and Obsessive-Compulsive Disorder (Rigoux et al., 2024; Conceição*, Petzschner* et al., 2023). Our research also examines how these cognitive processes interact with pharmacological and psychological therapies, offering insights that could lead to more effective treatment strategies (Conceição*, Petzschner* et al., 2023).